In our previous blog, we spoke about how we were able to apply our prototype Clio machine transcription capability to assist “scientists and researchers achieve time efficiencies and savings that they can apply to doing more good environmental work.” Gaia Resources also works closely with archives and museums across the country, and the same technology can be applied for a very different use-case there.
Our latest proof-of-concept activity with our valued clients at Queensland State Archives (QSA) has seen us helping to tap into the information stored in the archives. To do this, we used Clio to transcribe handwritten letters and historical documents that tell the story of the turbulent early contact between Australia’s First Nations people and European settlers in Queensland.
This work with QSA’s First Nations Access and Engagement Team is part of their Frontier Wars project that includes a significant collection of digitised 150 year old handwritten documents and letters, a community transcription initiative, an exhibition and a three-part SBS documentary series called Australian Wars. The documentary series premiered on Wednesday, 21 September, and it tells the story of Australia’s frontier conflicts that swept the country over a 100-year period.
When David and his team approached us with the challenge of transcribing 250 scanned handwritten letters and documents, we quickly jumped on the opportunity to be involved in such an interesting project. David was considering his options, including:
- Open community transcription, where volunteers manually transcribe documents through FromThePage software,
- Confidential online transcription, where the transcriptions are done manually by a small number of trained and certified professionals, and
- Automated transcription using Gaia Resources Clio prototype.
Using Clio for this project was an attractive option for transcribing sensitive handwritten documents quickly and efficiently; however, the varying cursive styles, condition of documents, formats and orientations of text presented several challenges. The benefit of Clio is that it is very fast to turn handwriting into a text document, so it can be easily scaled to hundreds and thousands of input documents.
The results from Clio can vary between getting just about every word correct with a few typos here and there, to only getting about half the content correct; and there are several factors that control that. As shown in the samples below, there are several variables to contend with, including blurred and crossed-out words, damaged pages, angled writing, newspaper formats and scans of books. Some of these require image pre-processing to get the best possible result.
David opted for a combination of automated and open community transcription, and worked with us to take the results from Clio as a starting point for more efficient community transcription in FromThePage software. We all knew that automation alone would not solve the problem, but Clio could help speed up the process. The idea of going forward with that combined approach was that if someone was to start from nothing, it might take them an average of 20 minutes to do a transcription; but with the Clio result as a starting point, they might only take 5-6 minutes to correct errors and move onto the next document.
What the QSA team found was that the Clio results – although variable – were better than they had expected, and were a great help in saving many hours of manually intensive work. Not only that, but enthusiastic members of the public enjoyed having a look at how the ‘machine’ had interpreted certain bits of text. In some cases, they could raise an eyebrow over something that is quite obvious to the human eye; but the efficiency gain becomes undeniable when scaled to a whole collection of documents.
Delving into our process and results a little, we were able to use a Clio-derived (e.g. computer) confidence in prediction value to categorise each document’s predicted transcription accuracy. Each term, word or text component in a document is assigned a confidence in prediction value based on how confident Clio is of its transcription for that component. The Average Confidence Level can then be calculated across the entire document.t.
Below are the Confidence Level categories used and their corresponding average confidence in prediction ranges:
- Very Good (between 90% and 100%)
- Good (between 80% and 89%)
- Above Average (between 70% and 79%)
- Average (between 60% and 69%)
- Poor (between 40% and 59%)
- Very Poor (between 0% and 39%)
Before we started, the QSA team suspected they might only get about 40% of the content accurately transcribed using Clio. The graph shows the percentage of documents that fell into each Confidence Level category.
We had no documents in the Very Poor category. So 100% of documents had an average confidence level exceeding 40%. Although it is only a computer derived measure, it hinted at how well Clio was coping with these historical documents.
This combination of automated and manual process saves time and enables people to get on with the important stuff that AI can’t do, and it follows that there are consistency and security benefits to go with a more efficient workflow.
Some other improvements we were able to implement in pre-processing the scanned documents included splitting ‘double page spread’ formats into separate pages. Below is an example of the inputs and outputs before and after splitting the pages:
While the statistical results of these may not have changed, the correctness and readability of the output is certainly better and important for the downstream review process.
As mentioned above, the condition of the original documents varied for a number of reasons. When it comes to documents with multiple orientations of text, Clio will only read one orientation per document n a single page, or it may merge text from adjacent blocks that are meant to be read separately – such as margin text or dates.
Future improvements could be gained with additional pre-processing by segmenting multiple text orientations, as illustrated below:
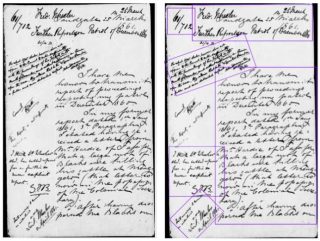
An example of multiple text orientations (left) and future approach of isolating blocks of text (right). (for illustration purposes only)
We also could do further post-processing as well as the pre-processing outlined above to improve results. For example, we could implement a method using the Levenshtein distance algorithm which would assist in finding similar strings in other documents and replace it with a more likely string. In this way we could correct ‘Social socuriy gumber’ to ‘Social Security Number’.
Overall, we were really impressed with the accuracy of the Clio prototype in reading 19th Century handwritten letters and folios. Clio’s models have been well-trained and are very good – not perfect, but very impressive and they are continually improving as more information is passed through it and the corrections are fed back in. Often the model is better at reading old handwriting than I am!
From our point of view, this has been an exciting Proof of Concept as it shows that it is possible and viable to unlock the vast and valuable information stored in archives, making the content more accessible and searchable to the general public.
If you’ve got data in a soft or hard copy format that you need transcribed, then reach out to us and let’s see how we can help you solve your problems. In the meantime, if you’d like to know more, start a conversation on our social media platforms – Twitter, LinkedIn or Facebook or send us an email.
Gail
Comments are closed.